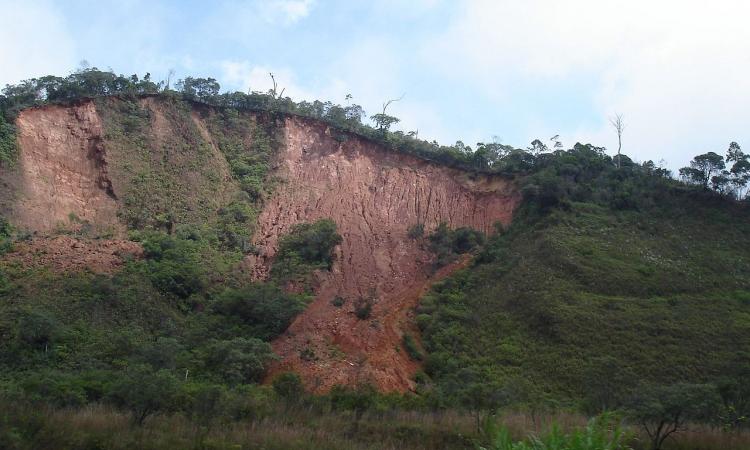
When landslides occur, their impacts are usually not discovered immediately beyond the attention of first responders or government agencies. Reliable spatial data becomes available to users based on the satellite return path and the route that it takes, image quality and processing time.
Further, landslides can be associated with rainfall or volcanoes meaning satellite data acquisition can be delayed due to poor image quality caused by cloud cover or whether the satellite passes the area in the day or night. Interpretation of these images also requires considerable effort by specialists although recent work aims to speed this process up using automatic image recognition.
This means that locating and assessing the disaster can take considerable time and most studies are based on post-event analysis. This time lag often ranging from days to weeks can affect emergency preparedness and disaster response.
A recent paper ‘A near-real-time global landslide incident reporting tool demonstrator using social media and artificial intelligence’ in the International Journal of Disaster Risk Reduction addresses the issue of or data latency, ranging from several hours to several days from when a disaster happens.
Social media data, while inherently imperfect, provide near-real-time information in large quantities and at spatial densities that may exceed conventional sensor networks that can complement data from other sources. These ‘social sensor’ data allow access to a rich source of human information such as text, videos, photographs, timestamps and coordinates and can report disaster information quicker than the observatories.
In 2021, there were 3.78 billion social media users worldwide and acquiring disaster data through these platforms has gathered pace, particularly over the last decade. While these data have great potential for near-real-time reporting of landslide events and their associated impacts, they are noisy and it is difficult for disaster managers to extract relevant and timely information.
The reporting of landslides and their impacts (damage and loss) varies widely across the globe reflecting a range of physical and socio-economic drivers and contexts and the attention of the mass media is not uniformly distributed across disaster-affected areas. This means that any attempt to quantify global landslide hazards and the associated impacts is an underestimation.
Few studies exist at present that provide a globally consistent set of estimates for landslide hazard. Landslides often occur in a multi-hazard cascading environment triggered by other more conspicuous, and therefore more widely reported, hazards such as earthquakes and tropical storms. Consequently, impacts such as the number of fatalities caused by landslides themselves are underestimated because they can be incorrectly reported as being the result of the trigger event, e.g., earthquake.
Historically, national and regional landslide databases have required substantial investment to enable the manual trawling of maps, aerial photographs, scientific papers, reports and the printed news media for data population.
Landslide data-gathering processes have changed considerably over the last two decades as digital technology, data availability, earth observation techniques, database standards and software interoperability have improved. The use of smartphones has increased the incidence, detail and speed of data reported where, in general, information (including photographs) of landslides is published on social media inherently because they have had an impact for humans of some kind. Indeed, dedicated citizen science smartphone applications have been developed specifically for hazard data capture by non-specialists.
To address these gaps, a landslide reporting tool using artificial intelligence (AI) that monitors social media in near-real time has been developed by scientists at the British Geological Survey, European-Mediterranean Seismological Centre, and Qatar Computing Research Institute.
The development of a system that monitors social media continuously for general landslide-related content using a landslide classification model to identify and retain the most relevant information is described and validated in the paper.
The researchers used machine learning to train the tool to identify landslides in photographs. For this, the geologists independently carried out an assessment of 11,737 images and manually marked each one as landslide or not a landslide.
The paper explores a well-known microblogging platform, Twitter, to identify landslide-related posts, specifically those with images containing landslides. A new methodology is presented that harvests landslide photographs from Tweets automatically and in real-time. To do this, different types of noise and irrelevant content that can be associated with landslide-related social media imagery data are identified. Moreover, a further aim is the annotation and release of a dataset for the community to develop image filtering and landslide detection tools.
This technical paper describes the underpinning theory and presents a detailed experimental approach to the model development step.
The tool extracts information from Twitter through keywords such as landslide, landslip, earth slip, mudslide, rockslide, and rock fall. The algorithm then looks for an image attached to the tweet. The system harvests photographs in real-time from these data and tags each image as landslide or not-landslide.
A training model was developed with input from computer scientists, geologists (landslide specialists) and social media specialists to establish a large image dataset that has then been applied to the live Twitter data stream.
The preliminary model was developed by training a convolutional neural network on the dataset. Quantitative verification of the system's performance during a real-world deployment shows that the system can detect landslide reports with a precision of 76%.
The demonstrator model is currently running live https://landslide-aidr.qcri.org/service.php; the next stage of development will incorporate stakeholder and user feedback. The tool is currently up and running and is intended to be used for disaster management, landslide research, climate change analysis, and global and national database studies.
The full paper can be viewed here